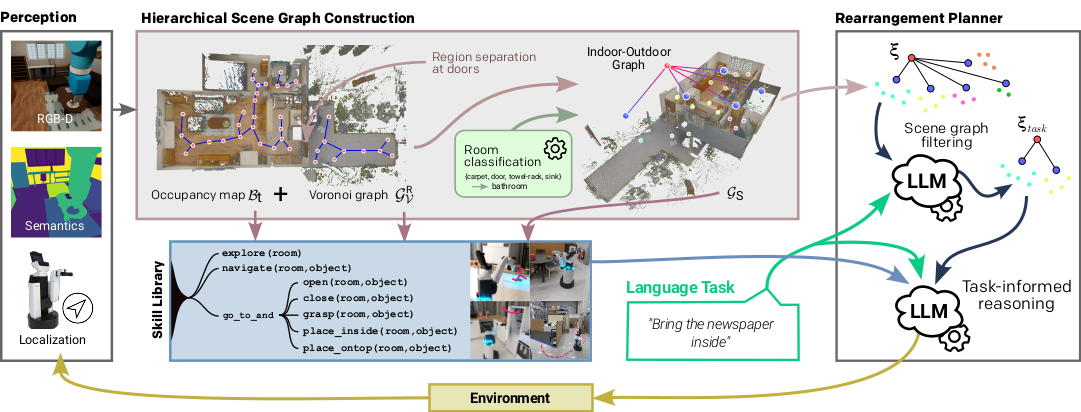
Autonomous long-horizon mobile manipulation presents significant challenges, including dynamic environments, unexplored spaces, and the need for robust error recovery. Although recent approaches employ foundation models for scene-level reasoning and planning, their effectiveness diminishes in large-scale settings with numerous objects. To address these limitations, we propose MORE, a novel framework that augments language models for zero-shot planning in mobile manipulation rearrangement tasks. MORE utilizes scene graphs for structured environment representation, incorporates instance-level differentiation, and introduces an active filtering mechanism to extract task-relevant subgraphs, thereby constraining the planning space and enhancing reliability. Our method supports both indoor and outdoor scenarios and demonstrates superior performance on 81 tasks from the BEHAVIOR-1K benchmark, outperforming existing foundation model-based methods and exhibiting strong generalization to complex real-world tasks.
This work is released under CC BY-NC-SA license. A software implementation of this project can be found on GitHub.
If you find our work useful, please consider citing our paper:
This work was funded by Toyota Motor Europe, an academic grant from NVIDIA, and the BrainLinks-BrainTools center of University of Freiburg.